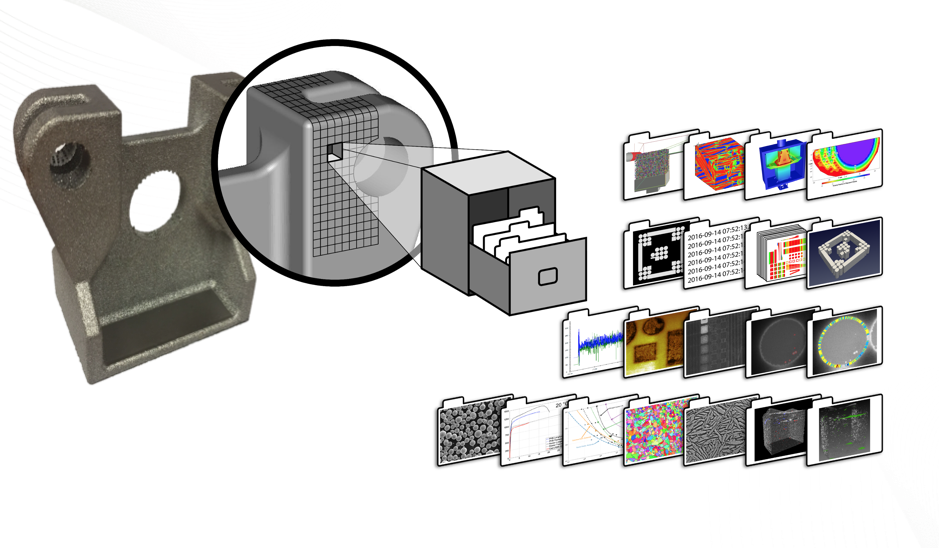
Few additively manufactured components are currently being used in production environments due to the challenges and costs associated with the certification and qualification of components. The current state of the industry is to certify components by using design allowables; an expensive method requiring fixed processing conditions.
ORNL in collaboration with industry, and other federal agencies, is developing a framework to collect, analyze, and interpret process and performance data of additive components. The framework is made of several software applications that can be used individually or applied as a whole to understand the condition of the additive component. The framework both accelerates learning about these new additive processes, and consistently captures pedigree data sets with associated process, property, and performance characteristics. Information captured includes process intent information, in situ process monitoring data, and post characterization information from both microstructure and mechanical performance. Statistical data analytics and visualization tools can then be used to determine correlations within the data, such as individual machine behavior over time, machine to machine variation, process performance relationships, and process conditions to defect and microstructure formation. As the framework matures, machine learning and uncertainty quantification will be applied to evaluate patterns that may be buried in the signals. Eventually, this technique will be used to develop closed loop systems that make real-time corrections to process parameters if the process is deviating from predetermined boundaries increasing the yield of performance-driven components and the reliability of additive manufacturing.
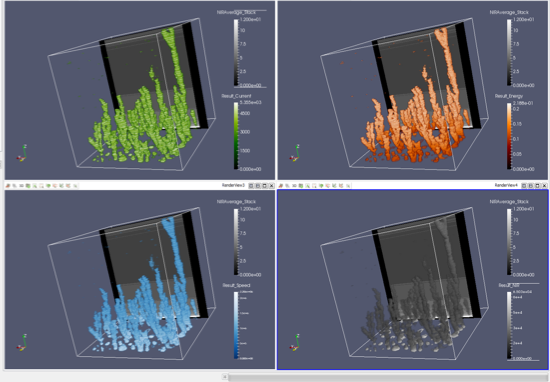
In addition, the unique and complicated thermal signatures associated with many additive manufacturing technologies typically result in non-homogeneous microstructures and non-uniform material properties. However, the advances in characterization techniques and developments in computational simulation can drastically accelerate the understanding of additive manufacturing process capabilities and limits.
ORNL is using integrated computational materials engineering (ICME) methodologies to accomplish this goal. Physics-based models are being used in connection with in situ sensors using non-destructive evaluation techniques and post processing characterization to accomplish this goal. ORNL is using sensors where available on systems or modifying equipment to use new sensors in order to evaluate the additive processes real-time and verify the conditions of the model. In addition, neutron imaging has also proven valuable in understanding the internal structure of additively manufactured metal components and to optimize functional performance.