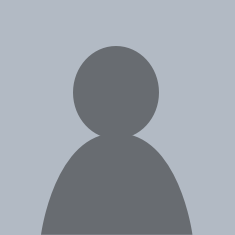
Bio
Dr. Minsu Kim is a research scientist at ORNL, specializing in the development of machine learning (ML) and artificial intelligence (AI) methodologies for healthcare analytics and diagnostics. In 2024, his work has centered on advancing AI-driven approaches for non-invasive cancer detection through spliceomic profiling of blood RNA, aimed at identifying early immune responses to cancer.
Dr. Kim has also led extensive studies leveraging electronic health records (EHR) to refine clinical pathways for mental health and cardiovascular conditions. His research includes analyzing psychotherapy patterns in veterans with major depressive disorder (MDD), uncovering pharmacotherapy treatment patterns, and developing standardized care guidelines for hypertension.
Dr. Kim's contributions have been widely recognized, including a publication in BMC Medical Informatics and Decision Making, with additional manuscripts under review and in preparation. He has also played a key role in organizing the 5th BTSD 2024 workshop at IEEE Big Data, fostering collaboration in AI and big data research.
Professional Experience
Research Scientist, Artificial Intelligence and Machine Learning
Oak Ridge National Laboratory (ORNL), Knoxville, TN
2023 – Present
Dr. Minsu Kim leads innovative projects at ORNL, focusing on AI-driven healthcare analytics. He has pioneered non-invasive cancer detection methods through spliceomic profiling of blood RNA, aimed at early identification of cancer-specific immune responses. His work with electronic health records (EHR) has refined clinical pathways for Major Depressive Disorder (MDD) and ischemic heart disease, improving personalized treatment strategies and standardizing care guidelines for hypertension. Dr. Kim also developed an NLP tool to enhance research precision by predicting relevant clinical literature. His leadership in organizing the 5th BTSD 2024 workshop at IEEE Big Data and mentoring junior researchers has advanced ORNL’s role in AI and healthcare research. His contributions are reflected in publications, including in BMC Medical Informatics and Decision Making, with more manuscripts in progress.
Postdoctoral Research Associate
Oak Ridge National Laboratory (ORNL), Knoxville, TN
2020 – 2023
At ORNL, Dr. Kim conducted AI and ML research focusing on biomedical data, including EHR and genomics. He developed predictive models and data-driven approaches to analyze clinical pathways and treatment patterns, contributing to significant advancements in personalized medicine.
Postdoctoral Researcher
Seoul National University, Bioinformatics Institute, Seoul, Korea
2019 – 2020
During his postdoctoral tenure at Seoul National University, Dr. Kim developed ML and AI techniques to predict cancer patient outcomes based on transcriptome data. His work contributed to the development of prognostic tools, enhancing the accuracy and reliability of cancer predictions.
Ph.D. in Bioinformatics
Seoul National University, Seoul, Korea
2013 – 2019
Dr. Kim earned his Ph.D. in Bioinformatics, where he laid the foundation for his expertise in computational biology and machine learning. His doctoral research focused on developing advanced algorithms to analyze large-scale genomic data, leading to significant insights in cancer research and the creation of novel bioinformatics tools. His contributions during this period included the development of SpliceHetero, an information-theoretic model for analyzing intratumor heterogeneity, which was published in PLOS ONE. Additionally, he contributed to the creation of predictive models for RNA-seq data, including tools for predicting lymph node metastasis in early oral cancer and recurrence in ER+ breast cancer patients, which were published in leading scientific journals.
Education
Ph.D. in Bioinformatics
Seoul National University, Seoul, Korea
2013 – 2019
- Dissertation: Focused on developing advanced machine learning algorithms and bioinformatics tools for analyzing large-scale genomic data, with significant contributions to cancer research. Key achievements include the development of SpliceHetero, an information-theoretic model for measuring intratumor heterogeneity, and predictive models for RNA-seq data applied to cancer prognosis.
B.E. in Computer Science and Engineering (Second Major)
Seoul National University, Seoul, Korea
2008 – 2013
- Acquired a strong foundation in computer science, with a focus on algorithms, data structures, and software development. This technical expertise provided the computational backbone for Dr. Kim’s later work in bioinformatics and machine learning.
B.S. in Biological Science
Seoul National University, Seoul, Korea
2008 – 2013
- Developed a deep understanding of biological systems, molecular biology, and genetics, which was crucial for integrating computational methods with biological data in subsequent research.
Professional Service
Organizer, 5th International Workshop on Big Data Tools, Methods, and Use Cases for Innovative Scientific Discovery (BTSD) 2024
IEEE Big Data Conference
2024
- Led the organization of the BTSD workshop, fostering collaboration and knowledge exchange among researchers in AI, big data, and healthcare analytics. This workshop brought together experts to discuss cutting-edge methodologies and their applications in scientific discovery.
Program Committee Member
IEEE International Conference on Bioinformatics and Biomedicine (BIBM)
2024
- Reviewed and provided critical feedback on submitted papers, ensuring the high quality of research presented at the conference. Contributed to the advancement of bioinformatics and biomedicine through active participation in the program committee.
Peer Reviewer
Journal of Biomedical Informatics
2024
- Conducted six peer reviews for manuscripts submitted to the Journal of Biomedical Informatics, contributing to maintaining rigorous standards in the field of biomedical informatics.
Mentor, RSI Intern Program
Oak Ridge National Laboratory
2024
- Mentored an intern on the project “Transformer-Based Encoding for Clinical Pathways,” providing guidance on AI methodologies and fostering the development of future researchers in the field.
Proposal Reviewer
Scientific Research Funding Proposals
2024
- Evaluated research funding proposals focused on enhancing Large Language Models (LLMs) for scientific research, contributing to the decision-making process for grant awards.
Trademarks and Patents
- Method for Predicting Prognosis of Breast Cancer
Korean Patent No. 10-2001153-0000 (July 2019)
Inventors: Yoon S., Kim S., Kwon S., Kim M., Kim H., Han H.- Developed a composition and method for predicting the prognosis of breast cancer, particularly in estrogen receptor-positive, HER2-negative patients, using next-generation sequencing data. This patent supports the clinical decision-making process regarding chemotherapy.
- Breast Cancer Prognosis Prediction Method and System Based on Machine Learning Using Next-Generation Sequencing
Chinese Patent No. 110070915A (July 2019)
Inventors: Ryu H., Ahn S., Lee J., Lee S., Lee H., Kim A., Kim C., Yoon S., Kim S., Kwon S., Kim M., Jo J.- Patented a machine learning-based system for predicting breast cancer prognosis, focusing on integrating next-generation sequencing data to enhance prediction accuracy and support personalized treatment plans.
- Breast Cancer Prognosis Prediction Method and System Based on Machine Learning Using Next-Generation Sequencing
Korean Patent No. 10-2017-0149834 (November 2017)
Inventors: Park I., Ryu H., Ahn S., Lee J., Lee S., Lee H., Kim A., Kim C., Yoon S., Kim S., Kwon S., Kim M., Jo J.- This patent details a comprehensive method and system utilizing machine learning techniques to predict breast cancer outcomes, offering a tool for clinicians to improve prognosis and treatment strategies.
Publications
MONTI: A Multi-Omics Non-negative Tensor Decomposition Framework for Gene-Level Integrative Analysis
Other Publications
- RDDpred: A Condition-Specific RNA-Editing Prediction Model from RNA-seq Data
BMC Genomics- This paper introduces RDDpred, a pioneering tool for predicting RNA-editing events from RNA-seq data. It has been recognized for its significant impact on genomic research, particularly for its ability to minimize false positives without requiring experimental validation. The work was presented at the Fourteenth Asia Pacific Bioinformatics Conference (APBC 2016), further underscoring its importance in advancing the field of RNA editing.
- SpliceHetero: An Information Theoretic Approach for Measuring Spliceomic Intratumor Heterogeneity from Bulk Tumor RNA-seq
PLoS One- This publication presents SpliceHetero, an innovative model for assessing intratumor heterogeneity through RNA-seq data. The approach has provided new insights into cancer progression and prognosis by analyzing spliceomic diversity within tumors, making a substantial contribution to cancer genomics research.
- A Secure SNP Panel Scheme Using Homomorphically Encrypted Data
BMC Genomics- The paper addresses the crucial issue of data security in genetic research by proposing a novel secure SNP panel scheme. It has been recognized for advancing methodologies that ensure the confidentiality of genetic data while maintaining analytical accuracy, making it a valuable resource in the field of genomics.
- Information Theoretic Sub-Network Mining Characterization of Breast Cancer Omics
Journal of Bioinformatics and Computational Biology- This research introduces a sub-network mining technique that characterizes breast cancer omics data, offering a new perspective on understanding the molecular interactions within cancerous tissues. The approach has been acknowledged for its potential to improve breast cancer diagnosis and treatment strategies.
- Protein Interaction Network (PIN)-Based Breast Cancer Survival Prediction
Methods- This paper presents a method for predicting breast cancer survival using protein interaction networks. The study has been praised for its innovative use of network-based approaches to identify critical biomarkers, offering a new tool for improving patient outcomes in breast cancer.
- Logic-Based Analysis of Gene Expression Data Predicts Pathway Crosstalk Between TNF, TGFB1, and EGF in Basal-Like Breast Cancer
Methods- This study develops a logic-based framework to analyze gene expression data, focusing on pathway crosstalk in basal-like breast cancer. The work is noted for its ability to elucidate complex molecular interactions, providing insights that could lead to more effective therapeutic strategies.
- Development and Validation of a Next-Generation Sequencing–Based Multigene Assay to Predict the Prognosis of Estrogen Receptor–Positive HER2-Negative Breast Cancer
Clinical Cancer Research- This publication details the development of a multigene assay using next-generation sequencing (NGS) to predict the prognosis of breast cancer. The assay has been validated for clinical use, offering a reliable tool for guiding treatment decisions in patients with estrogen receptor-positive, HER2-negative breast cancer.
- A Knowledge Network-Based Approach to Facilitate Annotation of Clinical Pathway Component Clusters
IEEE EMBS International Conference on Biomedical and Health Informatics (BHI)- Presented at a prestigious conference, this work introduces a knowledge network-based approach for annotating clinical pathway component clusters. The methodology enhances the ability to identify and analyze critical aspects of clinical pathways, contributing to more effective healthcare delivery.
- MONTI: A Multi-Omics Non-Negative Tensor Decomposition Framework for Gene-Level Integrative Analysis
Frontiers in Genetics- This paper proposes MONTI, a framework for integrating multi-omics data at the gene level using non-negative tensor decomposition. The approach has been recognized for its potential to uncover novel genetic insights and improve our understanding of complex diseases.