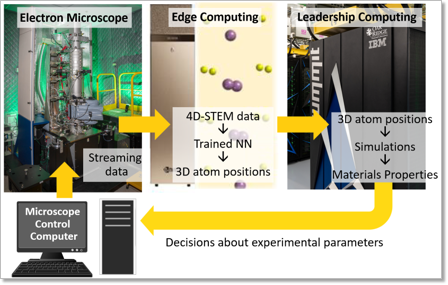
Over the last two decades, aberration-corrected scanning transmission electron microscopy (STEM) has become the mainstay of condensed matter physics, materials science, chemistry, catalysis, and nanotechnology. Yet both deriving fundamental physical insights from these multidimensional data sets and further using this knowledge towards making new and better materials for energy, quantum, and information technologies is stymied by the dearth of the methods and the data infrastructure necessary to handle the bespoke data sets. Similarly, the human-driven operation of microscopes is inherently limited in the age of fast data acquisition and multidimensional data. The purpose of this proposal within the INTERSECT framework is to establish the crucial link between the cutting-edge instrumental platforms at the Center for Nanophase Materials Sciences and High-Performance Computing capabilities at ORNL to enable the next generation of the HPC-driven scientific discovery. Specifically, the project will target three intertwined tasks, including (a) establishing a pipeline for direct data transfer from the STEM to the molecular dynamics (MD)/density functional theory (DFT) environment, (b) high-fidelity structural reconstructions from 4D STEM data, and (c) enabling the science-driven physical discovery via deep kernel learning. These targets, while closely linked scientifically, offer complementary challenges for the data infrastructure, prioritizing CPU-based calculations, GPU and high-volume data transfer, and low-latency GPU operations, respectively. Jointly, they will establish the INTERSECT framework capable of supporting the full spectrum of automated and big-data STEM operations across ORNL.