Inventing the future: AI for science
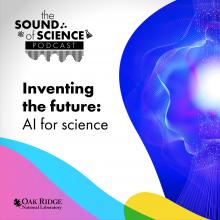
Artificial intelligence is no longer just a subject of science fiction. From personal assistants to self-driving cars, it's revolutionizing everyday life. While AI may be more prevalent these days, there are still a lot of misconceptions about what it is and what it isn't. In this episode you'll hear from a few of Oak Ridge National Laboratory's AI developers and practitioners. They'll dispel some of those myths and discuss how AI is revolutionizing cancer research, manufacturing and plant biology.
Transcript
[MUSIC/VOICES INTRO]
JENNY: Hello everyone and welcome to the Sound of Science – a brand-new podcast from Oak Ridge National Laboratory.
MORGAN/JENNY: We’re your hosts, Morgan McCorkle and Jenny Woodbery.
JENNY: Throughout the series we’re going to explore some of the stories behind the amazing science that takes place at ORNL.
MORGAN: Scientists and researchers at the lab will be joining us to discuss their groundbreaking work, from discovering new periodic table elements to fueling deep-space missions.
JENNY: We’re really excited to get to tell these stories and hope you’ll find them as fascinating as we do.
[MUSIC INTERLUDE]
MORGAN: Artificial intelligence. What comes to mind when you hear that term?
JENNY: Personal assistants? Self-driving cars? Sci-fi movies with robots that are going to take over the world?
MORGAN: Or all of the above?
JENNY: Alexa, what is artificial intelligence?
ALEXA: Is usually defined as the capacity of a computer to perform operations analogous to learning and decision making in humans.
MORGAN: Nothing like having an AI define AI. Alexa is correct – artificial intelligence is the ability for a machine to learn, reason and make decisions.
JENNY: But just because computers and machines can make decisions doesn’t mean they’re on a quest for world domination.
MORGAN: This is where a lot of the misconceptions about artificial intelligence come in to play. The sci-fi genre often shows AI becoming self-aware and overtaking human intelligence.
JENNY: However, what we’ve learn by talking to a number of the lab’s artificial intelligence developers and practitioners is that AI is only as good as the data it’s given.
KATIE SCHUMAN: There’s nothing magic about what’s happening. There isn’t some sort of magic leap and it suddenly has this intelligent behavior that emerges. We’re much less further along than I think the media portrays us to be.
MORGAN: That’s Catherine Schuman. She’s a computing researcher at Oak Ridge. Her work focuses on developing artificial intelligence, so we sat down with her to talk about what AI is and what it isn’t.
SCHUMAN: Artificial intelligence is a subset of computer science that is specifically looking for methods that learn from data, rather than explicitly programmed. Artificial intelligence is a broader term that encompasses many different techniques, including machine learning techniques as well as more statistical-based methods.
JENNY: We hear the terms machine learning and deep learning used a lot when discussing AI. But what is the difference between the two?
SCHUMAN: Machine learning is a particular subset of AI that deals with specifically learning from data directly. So, we are not programming any rules at all into how the machine learning system operates. These algorithms take the data and then they create their own rules of how they’re interpreting the data. It all comes from the data itself. Deep learning is a subset of machine learning that deals with specifically neural networks, which is a machine learning method that’s inspired by the way human biological neural networks process information.
MORGAN: Catherine’s AI research at the lab focuses on “nature inspired” machine learning, which includes looking at how the brain learns and processes information. So, in addition to computer science, she’s also exploring the field of neuroscience for her work.
SCHUMAN: We have a really nice, working example of something we know that can do all the things we want our machines to be able to do, and that is our brains. It’s our nice example to say “OK, that works,” let’s see if we can try to emulate what it’s doing so we can solve some of the problems we’re interested in. Particularly in science, there’s this explosion of data and we simply do not have the manpower to go through all of the data we’re interested in analyzing. We want to use machine learning in order to help us analyze this data more quickly.
JENNY: Pop culture would have us believe that machines can become instantly intelligent. However, in the real world, the amount of time it takes a machine to learn something can be a little surprising.
SCHUMAN: My favorite example is we used our algorithms to train a robot to navigate an unfamiliar environment with obstacles along the way. And we did this successfully and we can deploy it on the robot. But the amount of time it took for the robot just to turn left was kind of ridiculous.
JENNY: She said just teaching a computer to distinguish between cats and dogs is a challenge as well.
MORGAN: And because of this slow learning curve, there’s no need to be afraid of a robotic uprising any time soon.
SCHUMAN: I frequently get asked about the singularity, which is the point at which the computers over take human intelligence, and again we just figured out how to have them distinguish between cats and dogs, and remember that that network can only do cats and dogs and other animals, it can’t do anything else. So, it’s a long way to go before they overtake human intelligence.
JENNY: As machine learning methods are showing up more often in science and everyday life, Catherine says it’s important that people understand how they work so they can use them effectively.
SCHUMAN: We really want the whole population, not just computer scientists or scientists, to at least have a base understanding of machine learning and how AI functions, and what its limitations are. It isn’t just this magic, all-knowing system. It’s just math.
MORGAN: When it comes to using AI for science, ORNL has some pretty unique capabilities.
JENNY: For starters, the lab is home to the fastest supercomputer in the world, Summit.
MORGAN: At 200-petaflops, Summit can perform 200 quadrillion floating-point operations per second.
JENNY: So, if every person on Earth completed one calculation per second, it would take the world population 305 days to do what Summit can do in 1 second.
JENNY: And in 2021, the lab will get its first exascale system, Frontier, which will be more than five times faster than Summit.
MORGAN: This kind of tremendous compute power is ideal for handling massive datasets, which ORNL produces plenty of.
JENNY: The lab is home to several U.S. Department of Energy user facilities like the Spallation Neutron Source and the Center for Nanophase Materials Science.
MORGAN: These facilities allow scientists from all over the world to perform cutting-edge science with state-of-the-art instruments and technology.
JENNY: The experiments performed at these facilities produce tons and tons of data. Amounts of data that would take humans ages to sift through and analyze.
MORGAN: To leverage these assets and turn all of this data into science, ORNL established the AI Initiative.
JENNY: The AI Initiative brings together the lab’s AI expertise, computing resources and user facilities together to facilitate analyses of massive datasets that would otherwise be unmanageable.
DAVID WOMBLE: What the Artificial Intelligence Initiative is trying to do is develop a core AI research capability to support the transition from data into science. It’s kind of making a marriage, if you will, between our facilities and the scientific results that come out.
MORGAN: That’s David Womble. He leads the initiative.
DAVID WOMBLE: Almost every scientist here at Oak Ridge has incorporated some form of advanced data analytics, which is called machine learning, into their research. The question is now how do we move from that to the area where we are building out AI into a decision-making process.
JENNY: The decision-making component of AI allows research to go beyond simply analyzing data. It enhances the researcher’s ability to understand it and draw correlations that might have been missed by a human analyzing the data alone.
WOMBLE: I think it can do a lot to change what and how the scientist goes about their work. If you can imagine no longer being responsible for the drudgery of the lab, just day after day of literature survey and things like this, but something like that can help assimilate that information and lead toward the hypotheses.
MORGAN: Researchers from different areas at the lab are collaborating to harness the power of AI to solve complex problems in areas like advanced manufacturing, health care and even plant biology.
JENNY: ORNL is home to the Manufacturing Demonstration Facility, or MDF, where industry can collaborate with researchers to develop cutting-edge manufacturing techniques and tools.
MORGAN: Tom Kurfess has been working in the manufacturing field for more than three decades. He joined ORNL in 2018 as the lab’s chief manufacturing officer.
JENNY: He’s says AI is giving researchers the “big picture” of manufacturing.
KURFESS: Humans are great – they have their eyes, ears, they have all the senses. I can tell you when I work with some of my colleagues and we’re wiring something up, all the sudden you smell that burnt silicon and you say “uh oh, we wired something up wrong,” so you say you better unplug it. You bring all this information together from your sensory system. Now what AI can do is not just what I’m seeing or what I’m hearing, or even smelling, but over the entire production process, and I can bring all of that together for a much bigger picture. I see this as augmenting people, right? So, the people will never go away, but we’re going to get better at it and we’re going to use AI to help us move forward quickly.
MORGAN: Kurfess is leading the development of next-generation manufacturing platforms and implementing AI in processes like additive manufacturing, or 3D printing.
JENNY: A 3D printer builds parts layer by layer using materials like metal, plastic or even wood. With AI, researchers can gain extremely detailed insights into the structure and quality of the part.
KURFESS: AI allows you to make sure that you’re doing things right. It allows you to know that I just made this part and this part is good. On the process side, I can turn around and say “oh yes, this machine is running and I need it to be running for the next three, four or five weeks.” Yes, it can run for the next three, four or five weeks, or it can say I’m detecting a problem.
MORGAN: Kurfess says ORNL is an ideal location for industry partners to learn about implementing AI in manufacturing.
KURFESS: Applications for artificial intelligence, in particular the manufacturing area, Oak Ridge National Lab is the perfect place for it. We lead the nation; we lead the world in a number of different sectors of manufacturing. So why would you go anywhere else with your artificial intelligence to learn about the different processes we’re developing? The beauty about Oak Ridge National Lab, and in particular the Manufacturing Demonstration Facility, it is our objective to scale technology and get it out. So, it’s not just that we’re developing it, but this helps us develop it, make it useful for industry and get it out to our industrial partners so we can move the nation’s economy forward.
JENNY: Like in manufacturing, AI enables researchers in the biomedical field to quickly find and identify minute details in medical images.
JENNY: Gina Tourassi is the group leader for biomedical sciences, engineering and computing and director of the Health Data Sciences Institute at ORNL.
MORGAN: Gina’s work in AI is enhancing how physicians diagnose and treat cancer patients.
GINA TOURASSI: There are hundreds of thousands of women who are impacted by breast cancer on a yearly basis in the U.S. The screening tool of choice is screening mammography, which is an X-ray image of the breast. Very few people know that when it comes to the diagnostic interpretation of medical images, the diagnostic interpretation of screening mammograms is one of the most challenging problems for health professionals. It’s looking for a needle in a haystack.
MORGAN: While radiologists are highly trained to analyze mammograms, Gina said results can vary depending on who is reading them because of a person’s perceptual and cognitive differences.
TOURASSI: What my group has been doing is essentially leveraging AI to integrate the radiologists’ perceptual and cognitive behavior with the information from the medical image. Our technology has great potential to reduce the risk of medical error. Two human experts can read the same mammogram and make different errors for different reasons. So, we want to address the individual needs of the physician who reads, one case at a time.
JENNY: This is a real game-changer because it can provide truly personalized medical decision support for the physician and patient.
MORGAN: Gina says the role of AI in health care is to enhance the health care provider’s ability to serve their patients, not replace them.
TOURASSI: It’s not about replacing the individual, it’s about augmenting the individual in an intelligent way. AI is transforming health care delivery. However, I don’t expect to see anytime soon AI replacing physicians or nurses in day-to-day health care delivery.
JENNY: AI is even popping up in some pretty unexpected places, like ORNL’s greenhouses.
MORGAN: One of the lab’s greenhouses recently got a high-tech upgrade with an automated imaging system. To get an idea of what this looks like, imagine a conveyor belt system winding through a greenhouse. Plants are grown in pots along the conveyor belt and pass through an imaging station once a day.
JENNY: The station takes measurements of the plant’s observable traits, or phenotype. So, things like height, biomass and leaf shape are recorded.
MORGAN: This process will run 365 days a year and produce around 3.5 petabytes of data per year – or 3.5 million gigabytes.
JENNY: Julie Mitchell is the director of biosciences at ORNL. She and her team will employ AI to cull through the data collected.
JULIE MITCHELL: The data is so massive that you couldn’t possibly digest that as a human. You couldn’t make the associations; you couldn’t look at the sheer volume of data that comes out of the assembly line. So, AI is necessary in order to make those associations.
MORGAN: Julie said this will allow researchers to rapidly correlate the characteristics of the plants being studied and desirable traits like drought resistance and pest resistance.
MITCHELL: So that will also allow us to eventually take it out into the field and to see whether or not we need to apply different treatments, different fertilizer, different watering, or be able to identify if the crops in the field have a resistance to drought. If we can control the traits of plants through genetic engineering, which is guided by AI, we could potentially be growing plants on marginal lands, where we can’t currently farm.
MORGAN: Not only are ORNL researchers performing cutting-edge AI research, they’re also helping train the next generation to take advantage of AI in their own careers.
JENNY: Catherine Schuman does a lot of outreach work with middle school and high school students to get them excited about the field.
SCHUMAN: One of the interesting things about talking to middle school and high school students now is you can point to examples of where they’re interacting with AI and machine learning in their everyday lives. Whether that be on YouTube with the recommendations or asking Alexa or Siri to play a certain song. They have these examples in their everyday lives, so that gives them a starting point to understand, “OK, there’s something behind this that is making it work. What is it that’s making it work?”
MORGAN: During these events, students learn about how artificial intelligence works through hands on experience.
SCHUMAN: We even get to the point where they start messing with a bit of code and machine learning to show how easy it is to get started and to do something pretty intelligent, pretty quickly. We start with cats versus dogs as the typical example because people like cat pictures.
JENNY: As AI becomes more prevalent in science, having a working-knowledge of it will become a necessity.
SCHUMAN: AI won’t replace scientists and workers, but scientists and workers who use AI will replace those who don’t. It’s really important to understand how AI and machine learning methods work and what they’re limitations are in order to use them effectively.
MORGAN: So, as we’ve heard from our researchers, AI is changing the scientific landscape through its ability to make sense of massive amounts of data.
THOMAS ZACHARIA: People have referred AI, combined with data, as the oil of the future, if data is the oil, then platforms like Summit and Frontier are really the engines that transform oil into goods and services, and we are at the leading edge of that transformation.
MORGAN: That’s ORNL’s director, Thomas Zacharia. He sees Oak Ridge as uniquely quipped to lead advance AI for science as one of the Department of Energy’s national laboratories.
ZACHARIA: DOE generates a lot of amazing data at its user facilities. To be a leader you not only must have unmatched computing capability, but also you need unmatched data. And we have a collection of data particularly to drive science. Ultimately, it comes down to talented people. I don’t think there’s any other organization in the world that has access to as many talented people as DOE has across its 17 national laboratories. If DOE does not lead and drive this innovation, who will?
JENNY: From greenhouses to hospitals, AI is truly transforming every area of science at the lab.
ZACHARIA: I truly believe we are in the process of inventing the future, influencing how the future evolves. It’s going to have profound impact on how we live, how we work, how people are cared for, how you consume information; it’s an exciting time.
[MUSIC]
JENNY: Thank you for listening to this first episode of the Sound of Science. We’d love to hear your feedback in the comments section.
MORGAN: Don’t forget to subscribe so you get the latest episodes when they’re released.
JENNY: And if you want even more up-to-the-minute news from the lab, follow ORNL on Facebook, Twitter, Instagram and LinkedIn.
JENNY: Until next time!
[MUSIC OUTRO]